Introduction
In the tech and commercial worlds, artificial intelligence (AI) and machine learning (ML) , AI vs ML have become revolutionary technologies. These advanced technologies are transforming industries and redefining how we live and work. While often used interchangeably, There are clear distinctions between AI and ML. In this article, we’ll explore their core concepts, key differences, real-world applications, and both the benefits and challenges they bring.
What is Artificial Intelligence (AI)?
Artificial Intelligence, commonly known as AI, includes building machines that can simulate human intelligence. These systems are designed to think, learn, and do its duties without continual human assistance. The primary objective of AI is to develop technologies capable of reasoning, problem-solving, and replicating human cognitive abilities.
Understanding Machine Learning
Machine Learning (ML) is a specialized branch of AI that focusses on creating statistical models and algorithms that let computers learn from data. Unlike traditional programming, where explicit instructions guide actions, ML systems identify patterns in data and improve their decision-making capabilities over time, allowing for smarter and more efficient problem-solving.
AI vs. Machine Learning: A Comparative Analysis
Definitions
The key difference between Artificial Intelligence (AI) and Machine Learning (ML) lies in their core concepts. While machine learning (ML) is a subfield of artificial intelligence that allows machines to learn and grow from experience, artificial intelligence (AI) is the general concept of machines exhibiting intellect comparable to that of humans.
Scope and Applications
AI encompasses a wider range of domains, including robotics, computer vision, and natural language processing. It drives financial services, healthcare advancements, driverless cars, virtual assistants, and more. In contrast, machine learning (ML) is frequently utilised in fraud detection, recommendation systems, pattern recognition, and data analysis.
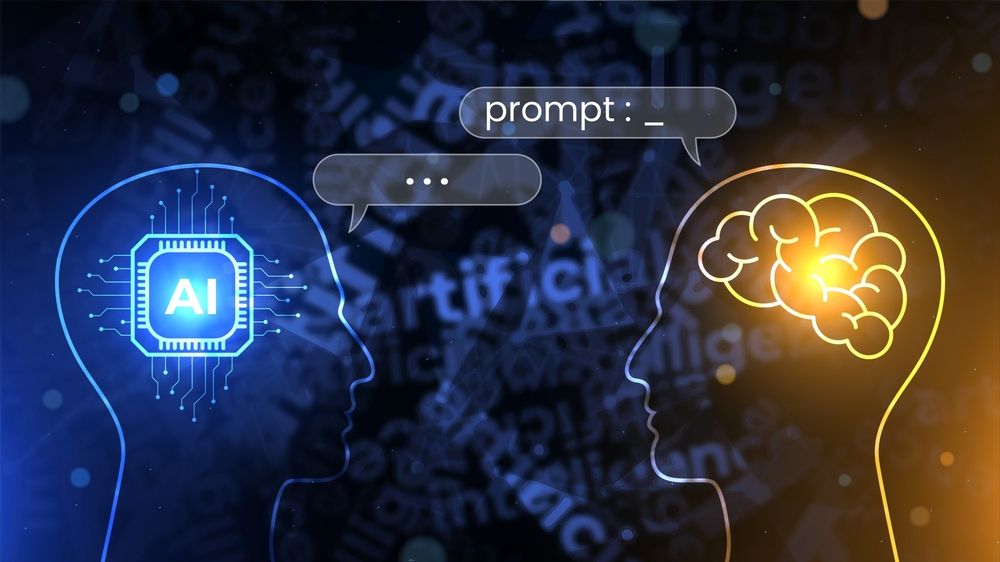
Learning Process
AI focuses on creating intelligent systems that can operate autonomously, whereas ML emphasizes training algorithms with data to enhance their performance through experience.
Human Intervention
AI systems can be either rule-based or self-learning. Some require human input to make decisions in uncertain scenarios, while others adapt independently. In contrast, ML heavily depends on human involvement during the training phase, where data is provided and algorithms are fine-tuned for accuracy.
Real-World Applications
AI is included in smart chatbots, self-driving cars, Siri, and Google Assistant, among other technologies. ML is behind personalized recommendations on streaming platforms, speech and image recognition, and credit card fraud detection.
Aspect | Artificial Intelligence (AI) | Machine Learning (ML) |
Definition | AI is the broader concept of machines that can simulate human intelligence, perform tasks, and make decisions. | Machine learning (ML) is a branch of artificial intelligence that allows machines to learn from data and perform better without explicit programming. |
Objective | The goal of AI is to create intelligent systems that can mimic human cognitive functions such as reasoning, problem-solving, and decision-making. | The goal of machine learning is to create algorithms that let computers identify patterns in data and make judgements based on that information. |
Scope | AI includes various technologies such as Machine Learning, Deep Learning, Natural Language Processing (NLP), robotics, and expert systems. | ML is a branch of AI and primarily deals with algorithms that analyze data and learn from it to improve predictions and decision-making. |
Approach | AI systems use a combination of rules, logic, decision trees, and learning models to perform tasks intelligently. | ML uses statistical models and algorithms to process data and adjust responses based on experience. |
Learning Process | AI is capable of operating both with and without data-driven learning. While some AI systems employ machine learning (ML) for ongoing development, others function according to preset principles. | ML is totally dependent on data. To train models that can classify or make predictions, a lot of data is needed. |
Data Dependency | AI systems may or may not depend on data. Some AI models operate based on expert systems and predefined rules. | ML is entirely data-driven, meaning the quality and quantity of data significantly impact its effectiveness. |
Decision-Making | AI systems aim to simulate human reasoning and can make decisions autonomously based on predefined logic or learned experiences. | ML models predict outcomes based on data patterns but do not inherently “reason” like AI systems. |
Human Intervention | AI systems can be fully autonomous or require human guidance, depending on the application. Some AI models function independently, while others need human feedback. | ML requires human intervention during training, where data is labeled, models are fine-tuned, and results are analyzed to improve accuracy. |
Examples | AI is found in virtual assistants (Siri, Alexa), autonomous vehicles, robotics, and AI-powered healthcare diagnostics. | ML is used in spam filters, recommendation systems (Netflix, YouTube), fraud detection, and speech recognition. |
Applications | AI is used in a wide range of fields, including robotics, expert systems, healthcare, self-driving cars, and intelligent automation. | ML is widely applied in predictive analytics, personalization, finance, marketing, and medical diagnostics. |
Flexibility & Adaptability | AI systems can be rigid if rule-based or highly adaptive if learning-based. | Because ML models are so adaptable, they can gradually increase accuracy by adjusting to new data. |
Processing Capability | AI can handle structured and unstructured data, integrating multiple sources of information to make complex decisions. | ML primarily works with structured and semi-structured data to extract patterns and make predictions. |
Advancements & Future | AI is evolving into General AI (machines that can think and reason like humans) and superintelligent systems. | ML advancements include deep learning, reinforcement learning, and self-supervised learning, pushing AI capabilities further. |
Artificial Intelligence vs. Machine Learning
Key Benefits of Artificial Intelligence
- Enhanced Efficiency: AI-driven systems can process information rapidly and with high accuracy, minimizing human errors.
- Task Automation: Automates repetitive and time-consuming tasks, enabling experts to concentrate on critical projects.
- Data-driven decision-making: AI is capable of real-time, large-scale dataset analysis to facilitate well-informed and effective decision-making.
Key Benefits of Machine Learning
- Predictive Analytics: ML models use historical data to forecast trends, helping businesses make proactive decisions.
- Adaptive Learning: By continuously learning from fresh data, machine learning algorithms improve their performance and accuracy over time.
Challenges of Artificial Intelligence
- Lack of Human Understanding: AI systems do not possess emotional intelligence, making it difficult to interpret human emotions and nuances.
- High Development Costs: Developing and maintaining AI systems may be expensive and time-consuming, requiring a large investment and level of experience.
Challenges of Machine Learning
- Data Sensitivity: ML models are highly dependent on data quality; biased or insufficient data can lead to inaccurate predictions.
- Overfitting Problems: Machine learning models may behave remarkably well on training data but poorly on fresh, untested data, which lowers reliability.
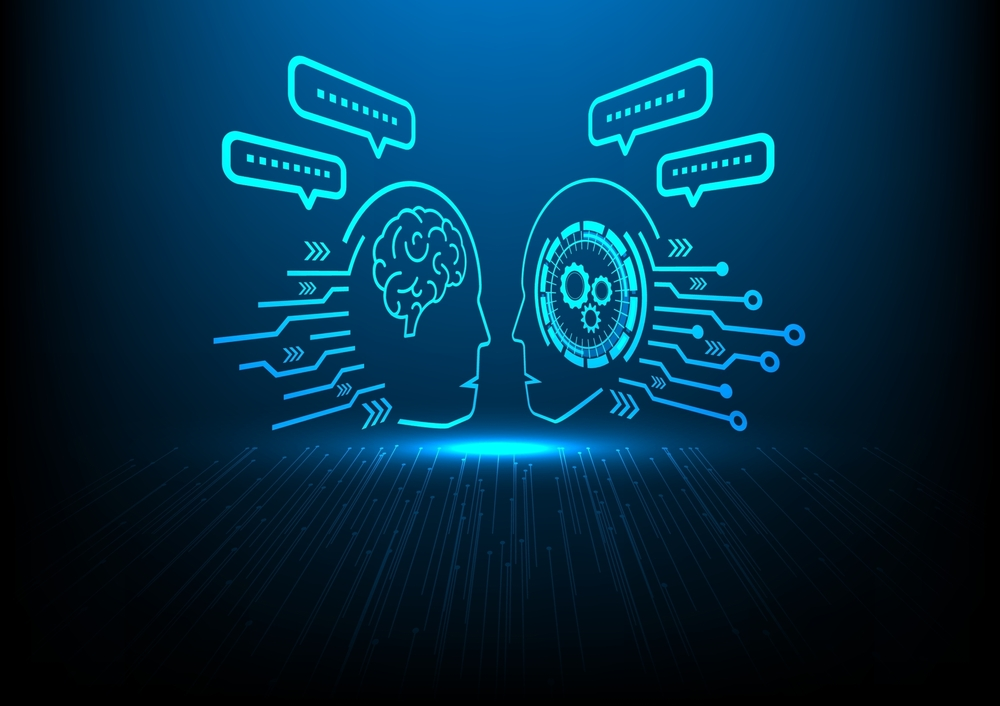
Future Trends and Innovations
Revolutionary developments in deep learning, natural language processing, and robotics are being made possible by the development of AI and ML. These innovations are set to reshape industries, revolutionizing human-technology interactions.
Ethical and Social Considerations
As AI and ML adoption grows, ethical concerns around data privacy, algorithmic fairness, and workforce displacement become increasingly significant. Long-term sustainability requires finding a balance between ethical use and technical advancement.
Conclusion
With their unique capabilities, machine learning and artificial intelligence are quickly changing a number of industries. AI focuses on building intelligent systems that simulate human thought, while Machine Learning enhances decision-making through data-driven learning. Both technologies bring unique advantages and face specific challenges, but together, they are fostering innovation across multiple fields. With the ongoing development of these technologies, their impact on automation, data analysis, and problem-solving will expand, driving significant advancements in technology and business. AI and machine learning have the potential to drastically change our environment in the future.