Mastering in Data Analytics Course in PUNE
What is data ANALYTICS?
Every day, businesses all around the world produce enormous amounts of data within form of log data, web servers, transaction records, and different customer-related data. Social networking websites produce a huge amount of data in addition to this.
In order to maximize value from their created data and make significant business decisions, companies should use all of it. This goal is driven by data analytics course in pune.
50+ hrs course duration
15+ hrs assignment duration
100% receive an Offer Letter
What will I learn about data analytics from this training?
Considering the explosion in the usage of analytics, enabling the company’s abilities to deal with data is not really valuable—practically it’s required. As more firms and industries join the bandwagon, the significance of these abilities will only increase in the future, which explains why there is currently such a concentration on data analyst course in pune.
Analytics is fundamentally a problem-solving tool. The issues simply exist on a far greater scale than most of us are accustomed to, impacting entire enterprises as well as the personnel and clients they serve. It’s always helpful to be able to think critically and tackle challenges in the appropriate manner.
The obvious advantage of understanding data analytics is this, and higher education students tend to concentrate on it the most. Simply put, data analysts are expensive, and with a growing shortage of skills on the horizon, this value will only grow as more firms and sectors start dealing with big data. In real life, this means that graduates with analytical abilities will be able to fetch higher pay and get first dibs on the available employment.
Graduates can gain financially from the increased demand for data analytics, but the big data boom too has resulted in a plethora of brand-new job prospects for skilled workers. This might be effective having the chance to work with a variety of various sectors, such as the government or the aviation industry, or simply being able to enjoy life.
As we’ve mentioned, the field of analytics is seeing a bit of a boom right now. With the wealth of data at our disposal today, the chance to use insight from such data is never higher. There will be a few effects, but the main one is that data analysts will become more valuable, leading to better work possibilities and options for professional advancement.
The beautiful thing about becoming an analytics expert is that the discipline entails far more than just being able to manipulate data and find solutions to issues. These conversational abilities are essential to any job, and the fact that they play a key role in a company’s decision-making processes means that analytics experts frequently develop great leadership abilities as well.
Advantages of Data Analytics
Organizational decisions are frequently based more on intuition than on facts and figures. One reason for this may be a lack of access to reliable data that can enable better decision-making. Analytics may assist in converting the available data into useful information for executives to enable them to make better decisions. If fewer bad decisions are made, this could be a competitive advantage since bad decisions can have a detrimental effect on a number of things, including organizational growth and profitability.
The time when a business could provide customers with a set of uniform goods and services is long gone. Customers want goods and services that really can cater to their specific requirements. Analytics may assist businesses in keeping track of the services, goods, or information that customers prefer and then presenting recommendations on their choices. Thanks to data analytics, customers can get services that are specific to their needs.
By identifying and fixing faults or preventing non-value-added tasks, data analytics can aid in improving the user experience. Self-learning systems, for instance, can use data to better understand how users interact with tools and make the necessary adjustments to enhance the user experience. Data analytics can also aid in automatic data cleansing, enhance data quality, and ultimately benefit both customers and enterprises.
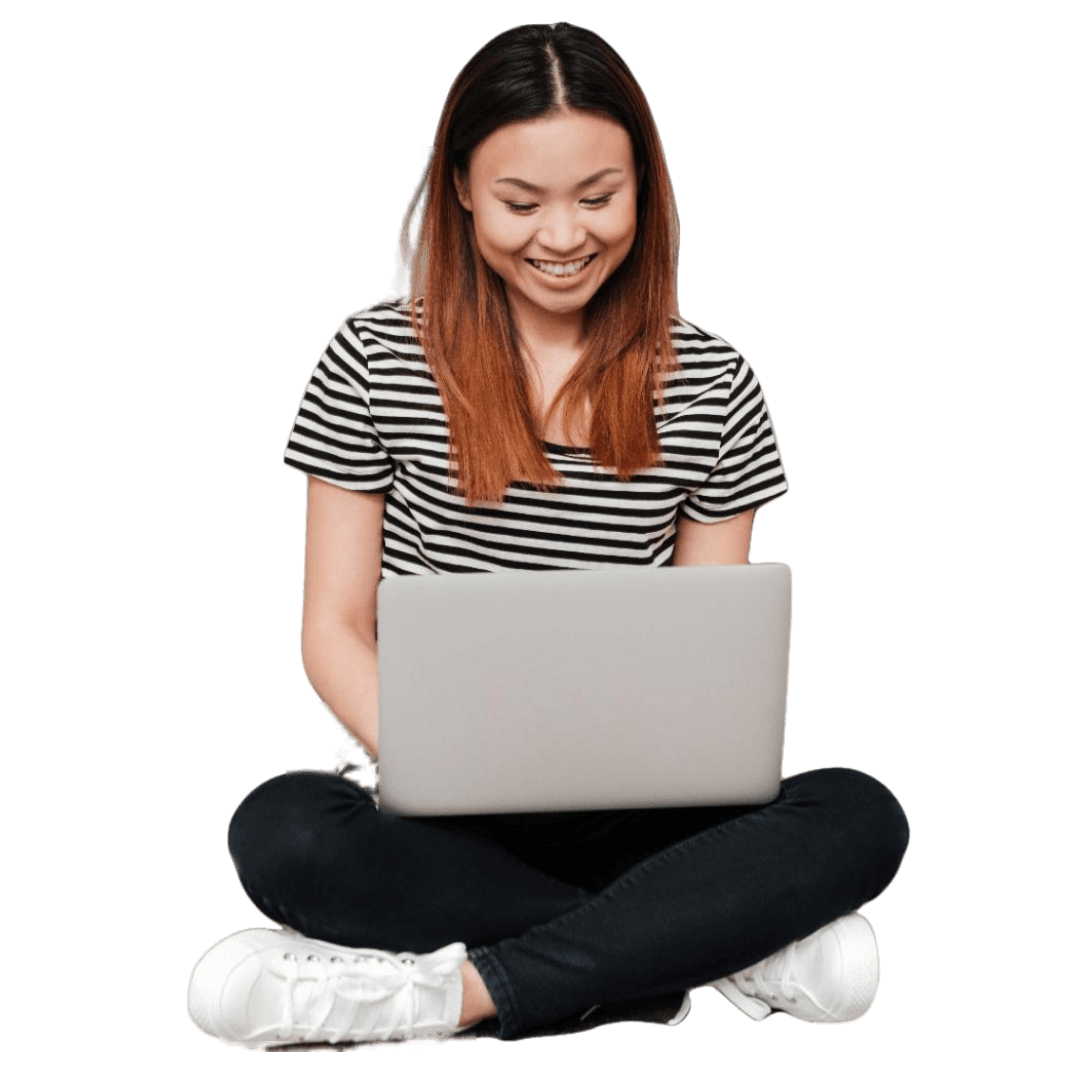
Mastering in Data Analytics Course in PUNE
In order to maximize value from their created data and make significant business decisions, companies should use all of it. This goal is driven by data analytics course in pune.
Discovering hidden patterns, and undetected trends, finding correlations, and gaining insightful knowledge from vast datasets are all part of the process of data analytics, which is used to create business forecasts.
Duration
6 months
Modules Covered
AWS, DevOps and Python
Assignments
Recordings
1 Year
Support
24X7
Study Material
Installation, setups, and eBooks
Placement Support
100% receive an Offer Letter
Calls
unlimited till 1 year
EMI Option
Available
Enquire Now
Brighten your career with Mastering in Data Analytics Course in PUNE
How to Get Started with Data Analytics
Start a Data Science career
- 5 Months of Class (or) Nine Months Online Specialized Placement Support
- By filling up the broucher provided by the university at reasonable data analyst course fees in pune.
Nothing stops you from Learning
Nothing stops you from learning, we provide EMI options for our trainings.
Apply Now
Mastering in Data Analytics Course Features
Job-oriented curriculum
Certified experienced Trainers
Lifetime access to study material
LIVE interactive online class
Flexible batch options
Reasonable fees
Software Installation Assistance
Hand-on training
Daily Assignments
Doubt Solving sessions
Industry-based projects
Missed class covered
Lifetime access to class recordings
Curriculum regularly updated
100% placement assistance
Soft skill workshop
Career counseling
Resume and LinkedIn profile preparation
Mock Interviews
Constant motivation and Guidance from instructors
Mastering in Data Analytics Course Syllabus
- Fundamentals of Data Science and Mathematical Statistics
- Introduction to Data Science
- Need of Data Science
- BigData and Data Science
- Data Science and machine learning
- Data Science Life Cycle
- Data Science Platform
- Data Science Use Cases
- Skill Required for Data Science
- Mathematics For Data Science
- Linear Algebra
- Vectors
- Optimization
- Theory Of optimization
- Gradients Descent
- Introduction to Statistics
- Descriptive vs Inferential Statistics
- Types of data
- Measures of central tendency and dispersion
- Hypothesis & inferences
- Hypothesis Testing
- Confidence Interval
- Central Limit Theorem
- Probability and Probability Distributions
- Probability Theory
- Conditional Probability
- Data Distribution
- Distribution Functions
- Normal Distribution
- Binomial Distribution
- An Introduction to RDBMS & SQL
- Data Retrieval with SQL
- Pattern matching with wildcards
- Basics of sorting
- Order by clause
- Aggregate functions
- Group by clause
- Having clause
- Nested queries
- Inner join
- Multi join
- Outer join
- Adding and Deleting columns
- Changing column name and Data Type
- Creating Table from existing Table
- Changing Constraints Foreign key
1. An Introduction to Python
- Why Python , its Unique Feature and where to use it?
- Python environment Setup/shell
- Installing Anaconda
- Understanding the Jupyter notebook
- Python Identifiers, Keywords
- Discussion about installed module s and packages
2. Conditional Statement ,Loops and File Handling
- Python Data Types and Variable
- Condition and Loops in Python
- Decorators
- Python Modules & Packages
- Python Files and Directories manipulations
- Use various files and directory functions for OS operations
3. Python Core Objects and Functions
- Built in modules (Library Functions)
- Numeric and Math’s Module
- String/List/Dictionaries/Tuple
- Complex Data structures in Python
- Python built in function
- Python user defined functions
4. Introduction to NumPy
- Array Operations
- Arrays Functions
- Array Mathematics
- Array Manipulation
- Array I/O
- Importing Files with Numpy
5. Data Manipulation with Pandas
- Data Frames
- I/O
- Selection in DFs
- Retrieving in DFs
- Applying Functions
- Reshaping the DFs – Pivot
- Combining DFs
- Merge, Join
- Data Alignment
6. SciPy
- Matrices Operations
- Create matrices
- Inverse, Transpose, Trace, Norms , Rank etc
- Matrices Decomposition
- Eigen Values & vectors
- SVDs
7. Visualization with Seaborn
- Seaborn Installation
- Introduction to Seaborn
- Basics of Plotting
- Plot Generation
- Visualizing the Distribution of a Dataset
- Selection color palettes
8. Visualization with Matplotlib
- Matplotlib Installation
- Matplotlib Basic Plots & it’s Containers
- Matplotlib components and properties
- Pylab & Pyplot
- Scatter plots
- 2D Plots
- Histograms
- Bar Graphs
- Pie Charts
- Box Plots
- Customization
- Store Plots
9. SciKit Learn
- Basics
- Data Loading
- Train/Test Data generation
- Preprocessing
- Generate Model
- Evaluate Models
10. Descriptive Statistics
- Observations, variables, and data matrices
- Types of variables
- Measures of Central Tendency
- Arithmetic Mean / Average
- Merits & Demerits of Arithmetic Mean and Mode
- Merits & Demerits of Mode and Median
- Merits & Demerits of Median Variance
11. Probability Basics
- Notation and Terminology
- Unions and Intersections
- Conditional Probability and Independence
12. Probability Distributions
- Random Variable
- Probability Distributions
- Probability Mass Function
- Parameters vs. Statistics
- Binomial Distribution
- Poisson Distribution
- Normal Distribution
- Standard Normal Distribution
- Central Limit Theorem
- Cumulative Distribution function
13. Tests of Hypothesis
- Large Sample Test
- Small Sample Test
- One Sample: Testing Population Mean
- Hypothesis in One Sample z-test
- Two Sample: Testing Population Mean
- One Sample t-test – Two Sample t-test
- Paired t-test
- Hypothesis in Paired Samples t-test
- Chi-Square test
14. Data Analysis
- Case study- Netflix
- Deep analysis on Netflix data
1. Exploratory Data Analysis
- Data Exploration
- Missing Value handling
- Outliers Handling
- Feature Engineering
2. Feature Selection
- Importance of Feature Selection in Machine Learning
- Filter Methods
- Wrapper Methods
- Embedded Methods
3. Machine Learning: Supervised Algorithms Classification
- Introduction to Machine Learning
- Logistic Regression
- Naïve Bays Algorithm
- K-Nearest Neighbor Algorithm
- Decision Tress
- SingleTree
- Random Forest
- Support Vector Machines
- Model Ensemble
- Model Evaluation and performance
- K-Fold Cross Validation
- ROC, AUC etc…
- Hyper parameter tuning
- Regression
- classification
4. Machine Learning: Regression
- Simple Linear Regression
- Multiple Linear Regression
- Decision Tree and Random Forest Regression
5. Machine Learning: Unsupervised Learning Algorithms
- Similarity Measures
- Cluster Analysis and Similarity Measures
6. Ensemble algorithms
- Bagging
- Boosting
- Voting
- Stacking
- K-means Clustering
- Hierarchical Clustering
- Principal Components Analysis
- Association Rules Mining & Market Basket Analysis
7. Recommendation Systems
- Collaborative filtering model
- Content-based filtering model.
- Hybrid collaborative system
- Artificial Intelligence
- An Introduction to Artificial Intelligence
- History of Artificial Intelligence
- Future and Market Trends in AI
- Intelligent Agents – Perceive-Reason- Act Loop
- Search and Symbolic Search
- Constraint-based Reasoning
- Simple Adversarial Search (Game- Playing)
- Neural Networks and Perceptions
- Understanding Feedforward Networks
- Boltzmann Machines and Autocoders
- Exploring Backpropagation
- Deep Networks & Structured Knowledge
- Understanding Sensor Processing
- Natural Language Processing
- Studying Neural Elements
- Convolutional Networks
- Recurrent Networks
- Long Short-Term Memory (LSTM) Networks
- Natural Language Processing
- Natural Language Processing
- NLP in Python
- Studying Deep Learning
- Artificial Neural Networks
- ANN Intuition
- Plan of Attack
- Studying the Neuron
- The Activation Function
- Working of Neural Networks
- Exploring Gradient Descent
- Stochastic Gradient Descent
- Exploring Back propagation
- Artificial and Conventional Neural Network
- Understanding Artificial Neural Network
- Building an ANN
- Building Problem Description
- Evaluation the ANN
- Improving the ANN
- Tuning the ANN
- Image Processing / Machine Vision
- Image basics
- Loading and saving images
- Thresholding
- Bluring
- Masking
- Image Augmentation
- Conventional Neural Networks
- CNN Intuition
- Convolution Operation
- ReLU Layer
- Pooling and Flattening
- Full Connection
- Softmax and Cross-Entropy
- Building a CNN
- Evaluating the CNN
- Improving the CNN
- Tuning the CNN
- Recurrent Neural Network
- Recurrent Neural Network
- RNN Intuition
- The Vanishing Gradient Problem
- LSTMs and LSTM Variations
- Practical Intuition
- Building an RNN
- Evaluating the RNN
- Improving the RNN
- Tuning the RNN
- Time Series Data
- Introduction to Time series data
- Data cleaning in time series
- Pre-Processing Time-series
- Data Predictions in Time Series using ARIMA, Facebook Prophet models.
GIT: Complete Overview
- Introduction to Git & Distributed Version Control
- Life Cycle
- Create clone & commit Operations
- Push & Update Operations
- Stash, Move, Rename & Delete Operations
Machine Learning Features & Services
- Using python in Cloud
- How to access Machine Learning Services
- Lab on accessing Machine learning services
- Uploading Data
- Preparation of Data
- Applying Machine Learning Model
- Deployment by Publishing Models using AWS or other cloud computing
1. Introduction to Data Visualization & Power of Tableau
- Architecture of Tableau
- Product Components
- Working with Metadata and Data Blending
- Data Connectors
- Data Model
- File Types
- Dimensions & Measures
- Data Source Filters
- Creation of Sets
2. Scatter Plot
- Gantt Chart
- Funnel Chart
- Waterfall Chart
- Working with Filters
- Organizing Data and Visual Analytics
- Working with Mapping
- Working with Calculations and Expressions
- Working with Parameters
- Charts and Graphs
- Dashboards and Stories
- Machine Learning end to end Project blueprint
- Case study on real data after each model.
- Regression predictive modeling – E-commerce
- Classification predictive modeling – Binary Classification
- Case study on Binary Classification – Bank Marketing
- Case study on Sales Forecasting and market analysis
- Widespread coverage for each Topic
- Various Approaches to Solve Data Science Problem
- Pros and Cons of Various Algorithms and approaches
Why choose us?
All of our online activity, including texts, emails, tweets, user inquiries (on results pages), social network exercises, and data generated by IoT & connected devices, is covered by big data. The enormous and complex number of information generated again by the digital world cannot be handled by traditional statistical information analyst classes in pune technologies. data science and analytics.
Since Big Data, Information Science, & Data Analytics are also still evolving technologies, data analytics classes in pune generally employ Data Science and Data Analytics interchangeably. The fundamental reason for the misunderstanding is that both data scientists and data analysts use big data in their job. The significant distinctions between data analysts & data scientists are what fuel the debate between data science & data analytics.
- Candidates with 0–3 years of job experience will do best.
- Candidates who are currently enrolled in their last semester of undergraduate study are also eligible to apply.
Career Opportunities for Data Analytics
Opportunities for Careers in Data Analytics : Data analytics are more critical than ever in the current digital era. With the growth of data analytics specialists rising daily, there are several job opportunities across numerous industries. The following list includes a few career options that call for data analytics specialists:
Data scientists gather and examine this data in order to use data visualization to make the appropriate conclusions. A data scientist must have a broad understanding of data, be proficient in data analytics & data visualization, and have programming language experience in languages like SQL, Python, Java, etc.
A data scientist makes an annual income of Rs. 634,645 on average.
Data engineers support the creation, use, and improvement of data infrastructure to support the different data analytics operations. A data engineer typically works with relatively sizable data sets and frequently contributes to data profiling and cleaning to make the data legible for data scientists.
A Data Engineer typically earns Rs 809,923 a year.
A business analyst uses data analytics to comprehend business models, corporate reports, technology integration documentation, etc. and offers various business solutions to help solve the business difficulties that an organization is facing.
Business analysts make an average of Rs 588,313 annually.
To provide coherent and helpful information, statisticians gather, analyze, and interpret statistical data. Among the regular tasks of statisticians are provide mathematical modeling, interpretation, and analysis of various results of the survey, statistical simulations, business forecasts based on data analytics, etc.
A statistician typically earns Rs 475,370 annually.
A computational intelligence engineer uses data analytics to analyze and evaluate statistical models and machine learning algorithms. For a data scientist, knowledge in both programming & statistics is primarily needed.
A computational intelligence engineer makes an annual salary of Rs 705,035.
A quantitative analyst uses data analytics to examine vast amounts of data in order to comprehend financial management, investment patterns, trending currencies, the stock market, etc.
A quantitative analyst makes an average of Rs. 842,849 per year.
These are all just a several of the industries that require data analytics. However, data analytics courses in pune with placement as a whole is a huge industry with countless prospects. With even greater growth anticipated in the future, there are many other employment options and chances within the data analytics industry. Therefore, a profession in data analytics is indeed a lucrative option with tremendous potential for future growth.
FAQ
Frequently Ask Questions.
Frequently asked questions for DevOps Online Training