Introduction
Many data scientists still use Excel for a variety of tasks, even in the face of the growth of specialist data science platforms. Microsoft Excel is another tool that is still very relevant in data science, even if it is frequently linked to Data science course in Pune for cutting-edge tools like Python, R, and SQL.
Importance of Advanced Excel in Data Science
Professionals in the field of data science rely on Advanced Excel to efficiently analyze, modify, and visualize data. In straightforward terms, let’s examine how advanced Excel abilities advance the field of data science.
Data scientists can successfully analyze, modify, and visualize data with the help of Advanced Excel. Professionals can maximize the value of their data and promote well-informed decision-making by managing big datasets, carrying out intricate computations, and producing intricate visualizations
Overview of Excel
Microsoft Excel is a spreadsheet application that offers tools for data organization, analysis, and visualization. Since its initial release in 1985, it has remained a mainstay in numerous industries thanks to its user-friendly interface and adaptable capabilities. Users may now perform more complicated data jobs than ever before because to Excel’s tools, which include pivot tables, VLOOKUP, and more recently, Power Pivot and Power Query. Regardless you can write programs or not, Excel is the most useful tool for altering and displaying data.
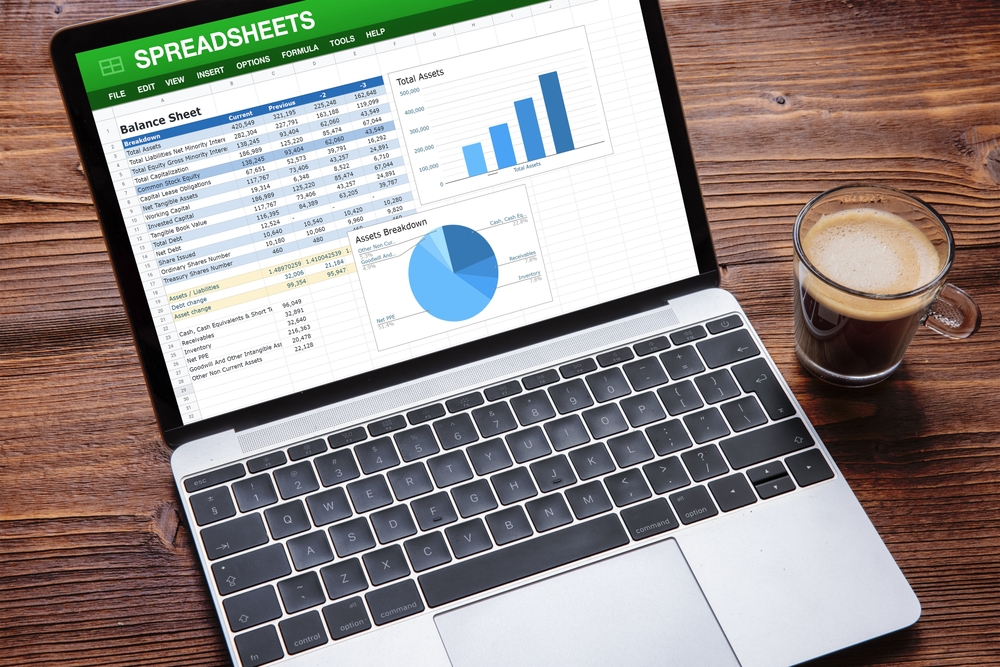
Why Data Scientists Use Excel
- Usability: Excel is accessible for rapid, exploratory data analysis since it is easy to use and doesn’t require a deep understanding of programming. Rapid data slicing and dicing with little effort is made possible by interactive features like pivot tables. If everything can be done in Excel without the need for programming, that would be great.
- Knowledge and Pervasiveness:Ideal for: Experts in a range of fields, such as operations, marketing, and finance, where it’s critical to analyze data quickly without requiring a deep understanding of code. Not appropriate for: Jobs requiring sophisticated statistical analysis or large datasets.
- Platform for Cooperation: Excel files are frequently utilized as a shared platform for department-level collaboration because they are simple to distribute. In companies where non-technical employees must access and evaluate data, it is very helpful.
- Efficient Validation of Data: Before using more powerful data science tools, Excel is frequently utilized for rapid data validation. It acts as an initial assessment of the consistency and quality of the data.
- Visualization:Excel has enough fundamental visualization features for basic charts and graphs, but it is not as strong as specialized programs like Tableau.
Role in Data Science
What-If Examination:
Data scientists can examine several scenarios and evaluate how modifications would affect results by using Excel’s “What-If Analysis” tool. Making decisions based on data-driven insights is made easier with this feature.
Analysis of Statistics:
Excel has built-in tools and functions for analyzing data statistically. Among other methods, data scientists can perform regression analysis, generate histograms, and compute descriptive statistics to extract insights from the data.
Data manipulation:
Data scientists can easily clean, transform, and work with huge datasets using advanced Excel. To get data ready for analysis, they can do things like sort, filter, and summarize it.
Data Visualization:
To assist data scientists in seeing patterns and trends in the data, Excel provides a number of visualization tools, such as pivot tables, graphs, and charts. Findings and ideas are easier to convey to stakeholders when presented visually.
Advanced Excel Techniques
Professionals who want to thrive in data science with thrive need become proficient in a number of sophisticated techniques, like as
Data Modeling:
Users can perform multidimensional analysis and establish associations between various datasets using Excel’s data modeling tools. This enables more advanced data reporting and analysis.
Advanced Formulas and Functions:
Excel provides a vast array of sophisticated formulae and functions for carrying out intricate computations and data analysis activities. Proficiency in complex functions such as array formulae, VLOOKUP, and INDEX-MATCH can greatly improve data analysis skills.
PivotTables and Pivot Charts:
The tools PivotTables and Pivot Charts enable users to rapidly summarize and examine massive datasets. PivotTables are capable of combining data according to various criteria, and Pivot Charts offer visual representations of the data that has been condensed.
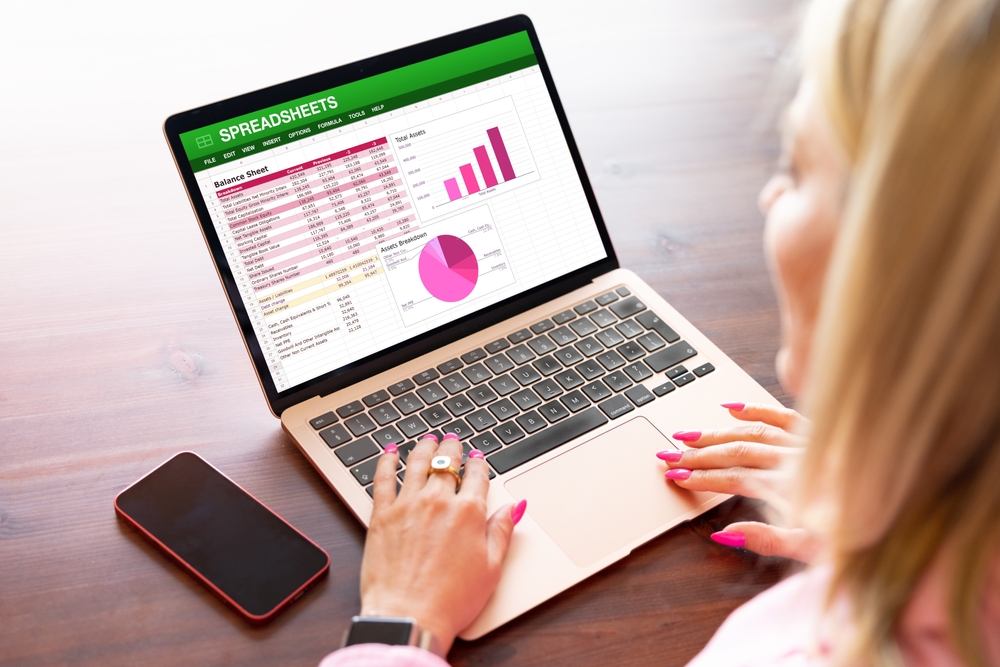
Comparative Analysis: Excel vs. Advanced Data Tools
Excel’s simplicity is both one of its advantages and disadvantages. This is how it stacks up against more sophisticated tools:
R:
Suitable for:
statistical calculations and in-depth data analysis using functionality-extending packages.
Unsuitable for:
activities involving fast data manipulation that profit from a graphical user interface.
Complementary Method:
For extensive statistical modeling, R may be utilized; the results can then be transferred to Excel for display.
Python
Suitable for:
more complex tasks, such as statistical analysis, machine learning, and managing big datasets because of the library’s broad support.
Unsuitable for:
circumstances in which end users who lack programming knowledge must interact with data.
Additional Function:
Data processing can be handled using Python’s Pandas module and then exported again to Excel for visualization.
Use Cases and Real-World Applications
In many areas, Microsoft Excel is still a widely used data science tool.
Data Validation and Business Reporting
With its ability to easily create pivot tables and graphs from CSV information, Excel continues to be a choice for business reporting.
Statistics and Data
According to a Market Research Future (2023) analysis, Excel will play a major part in the global market for business intelligence tools, which is predicted to increase by 11.1% between 2023 and 2030 because of its extensive and long-standing use in the business sector.
Compatibility of Programming Languages
Excel’s integration of programming languages is particularly helpful when working on more extensive data science projects or doing more intricate analysis.
A Visual Method for Managing and Organizing Data
The way that information and data are represented in programming languages and data science tools can be heavily text-based. It can be challenging to view or visually interpret some datasets, particularly when working on a big data project.
Key Takeaways
Excel’s continuing versatility and the continuous need for user-friendly data manipulation tools are major reasons for its prolonged popularity in the data science field. Excel’s widespread use in Advanced Excel, SQL, Python Libraries, Tableau, Advanced Machine Learning, and Deep Learning algorithms to solve day-to-day industry data problems in healthcare, manufacturing, sales, media, marketing, and education sectors making you job-ready for 30+ roles.
In nutshell, Excel is still a vital component of many data scientists’ toolkits, even though it might not be the best choice for every data science work. Its widespread use across numerous industries is guaranteed by its simplicity, ease of use for fast data checks, and capacity to display data in an understandable manner. Excel provides data scientists with an easy-to-use and effective way to interact with data, complementing more sophisticated tools. Data science course in Pune can select the best tool for the job by utilizing both conventional and contemporary data science solutions, guaranteeing accuracy and efficiency in data processing and analysis.